Volumez's Data Infrastructure as a Service platform helps organizations maximize GPU utilization and automate AI/ML pipelines while reducing costs in the cloud.
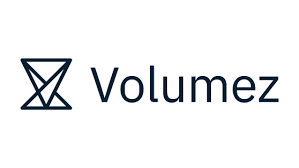
As enterprises rapidly increase their AI investments, with global spending expected to reach $500B by 2027, according to IDC, many organizations are discovering that traditional infrastructure approaches create significant bottlenecks in their AI/ML pipelines. Volumez, a Silicon Valley startup, is tackling this challenge with a novel "Data Infrastructure as a Service" (DIaaS) platform that promises to maximize GPU utilization while dramatically reducing costs.
During a 60th IT Press Tour presentation, Volumez executives explained that the fundamental problem lies in the inherent imbalance of current cloud infrastructure configurations. This imbalance manifests in several ways: I/O bottlenecks, storage inefficiencies, underutilized GPUs, complex management requirements, and data scientists spending excessive time on infrastructure instead of model development.
"The complexity of the cloud is not serving its consumers," explained John Blumenthal, Chief Product & Business Officer at Volumez. "It's beyond human cognition to correctly assemble the right instance with the right network configuration, with the right storage in the right physical locations, to meet the criteria that my workload requires."
The Volumez Solution
Rather than acting as a traditional storage company, Volumez provides a SaaS platform that configures and optimizes cloud infrastructure components. The platform's key innovation is its "cloud awareness" - a deep understanding of cloud provider capabilities and constraints that enable it to create perfectly balanced systems for specific workloads.
The company's approach differs from traditional storage controllers in using a lightweight user-space process that receives intelligence from the Volumez SaaS service. This "configurator" automatically creates optimized data paths using standard Linux components without introducing proprietary elements into the data path itself.
Impressive Performance Results
In recent MLCommons MLPerf Storage 1.0 benchmarks, Volumez demonstrated impressive results:
1.14 TB/sec throughput
9.9M IOPS
92% GPU utilization
411 simulated GPUs
These results significantly outperformed traditional storage approaches while delivering substantial cost savings - showing improvements of 27-70% in storage costs and 50-92% in compute expenses compared to standard AWS configurations.
Focus on Data Scientists
A key benefit of the Volumez approach is simplifying infrastructure management for data scientists. Integrating with tools like PyTorch allows data scientists to specify their infrastructure requirements directly from their notebooks without having to coordinate with ML ops teams.
"Having PhDs create the infrastructure and deal with the infrastructure is a recipe consistently delivered with waste and the inability to achieve what you set out as a data scientist to achieve," noted Blumenthal. "That's why many of these projects fail - the data scientist is doing janitorial work."
Market Focus
While Volumez's technology could potentially benefit many workloads, the company is initially focusing on AI/ML training workloads with specific characteristics:
Large data samples (medical imaging, video, audio, time series)
Multi-node training requirements
Capacity requirements that vary with GPU count
The solution appears particularly valuable for organizations in sectors like:
Medical imaging (CT/MRI analysis)
Autonomous vehicles
Media & Entertainment
Security & Defense
Financial services
Expert Validation
Dr. Eli David, a prominent AI researcher and advisor to Volumez, emphasized the critical nature of the problem the company is solving: "For many of the state-of-the-art models that I'm training at the moment, I'm not getting 100% GPU utilization... 50% utilization of a GPU just means that I'm paying double of what I would have liked to pay for my GPUs."
Future Implications
The Volumez approach could have significant implications for the economics of AI model training. By maximizing GPU utilization and automating infrastructure management, organizations can potentially:
Reduce time-to-model deployment
Lower total infrastructure costs
Free up data scientists to focus on model development
Improve model quality through faster iteration
Looking Forward
As AI workloads grow in size and complexity, especially with the rising adoption of generative AI, the need for optimized infrastructure solutions becomes increasingly critical. Volumez's cloud-aware approach to infrastructure optimization presents an interesting alternative to traditional storage-centric solutions.
The company's focus on automation and simplification, combined with its impressive performance metrics, suggests it could play an essential role in helping organizations overcome the infrastructure challenges that currently limit AI adoption and effectiveness.
For organizations struggling with AI infrastructure challenges, particularly those running large-scale training workloads in the cloud, Volumez's DIaaS platform merits consideration as a potential solution for reducing costs while improving GPU utilization and data scientist productivity.
Comments